Why sustainable AI Is a win-win-win: Photoroom’s path to greener, faster, smarter AI
The rise of AI raises major energy challenges. However, technical optimizations are already reducing carbon footprints while improving performance and costs.
The role of AI in our society is evolving, and so is its carbon footprint. A Goldman Sachs study shows that AI’s energy consumption is expected to increase by 2.5 times by 2030. Sam Altman, CEO of OpenAI, stated at Davos that the future of AI depends on an energy breakthrough. So, should we make no effort while waiting for this so-called breakthrough?
This represents a missed opportunity because, in AI, it is possible to combine sustainability, cost reduction, and improvement of the user experience. Photoroom experienced this in 2023, with its recurring annual revenue (ARR) doubling in just one year, from $20 million in ARR at the end of 2022 to $40 million in ARR at the end of 2023. In 2024, we are reaffirming our commitment by switching to renewable energy for the training phase of our models, along with new algorithm improvements in terms of computation time and generation quality.
These decisions highlight the importance we place on limiting the environmental footprint of our AI. With clients like Amazon, Depop, Decathlon, and Doordash, Photoroom’s AI processes over 2 billion images per year. At this scale, every improvement matters. As a global leader, we hope to inspire other players in the ecosystem to do the same.
The win-win-win of sustainable AI
In most industries, investing in sustainability is an additional effort, often outside the company’s business model. However, in AI, this is not the case: working on the sustainability of AI is actually a win-win-win, where companies can simultaneously improve the user experience, reduce costs, and minimize their environmental impact. For example, after reducing its execution time by 100 and improving the quality of its AI models, Photoroom saw its revenue double, to reach $50 million Annual Recurring Revenue (ARR) in 2023. By optimizing its models, Photoroom was able to provide faster and more relevant results, leading to better user engagement, while also contributing to cost reduction and lowering CO2e emissions.
Here’s how this was achieved:
Improving the user experience: By reducing the time needed to process images — from 2 minutes to 2 seconds — and improving the quality of the generated image, our users were able to get professional-level results quickly with a smoother user experience. Following these changes, our users showed massive adoption of Photoroom, especially in the e-commerce sector.
Reducing costs: Photoroom processes over 2 billion images per year. Optimizing processing times allows us to use fewer computing resources (GPU), significantly reducing operational costs. Thanks to a more efficient AI, it becomes possible to serve more users while keeping operational costs low. This operational efficiency is a key factor in Photoroom's rapid growth.
Reducing CO2 emissions: By reducing the time needed to generate results, Photoroom minimizes the computing resources used and the electricity required for each query, leading directly to a reduction in CO2 emissions. Generating a new background for your product photography with Photoroom uses less energy than 30 seconds of LED light and emits CO2 comparable to three human breaths—far less than a phone charge.
These points highlight that efforts toward sustainable AI can go hand in hand with improving the user experience and reducing operating costs.
Why AI consumes energy
To understand how AI impacts the environment, it is essential to break down how AI consumes energy. The energy needs of AI technologies primarily come from two stages: training and inference.
Training:
The training phase is comparable to building a car: it is energy-intensive because it involves processing vast amounts of data to refine and adjust the parameters of the AI model. This phase can last weeks or months, with powerful GPUs running continuously and consuming significant energy. The complexity of the model, the amount of data processed, and the training duration all contribute to the overall energy consumption. During this phase, servers run nonstop to process the data.
Inference:
After training, the AI enters the inference phase, where it processes user queries or generates outputs. This phase is comparable to driving the car: each query that the AI responds to consumes energy. As AI adoption and usage increase, the volume of inference queries grows, leading to a proportional increase in energy demand. The energy consumption during inference is particularly significant for AI companies with large user bases, with millions or even billions of queries processed every year.
Sustainable developments in AI
Companies committed to sustainability in AI can take concrete steps to optimize both the training and inference phases to reduce energy consumption and minimize their carbon footprint.
Optimizing training:
In 2024, we switched of cloud provider and chose Genesis Cloud, a renewable energy provider powered by hydroelectricity. More than 1000 tons of CO2e per year, equivalent to the emissions of 1000 flights between Paris and New York, are now produced by renewable energy rather than carbon-based energy. By choosing green energy, AI companies can drastically reduce their carbon footprint, even at the training stage.
Optimizing inference:
Photoroom’s inference runs on Google Cloud servers, capable of handling the high volume of queries we process — over 2 billion images per year — one one of their the lowest carbon impact data center. Google’s carbon-free energy (CFE) score in this location is 95%, ensuring that the percentage of carbon-free energy purchased at a local and hourly level is primarily renewable. Photoroom also presented inference optimizations at the Nvidia NGC 2024 event, which resulted in a 25% reduction in latency. Additionally, distillation reduced the number of processing steps required, from 20 to 6, achieving a 3x reduction in latency, contributing to better performance and lower energy consumption.
Developing specialized models:
Specialized models are designed to handle specific use cases, rather than attempting to address a broad range of tasks. These models typically require fewer parameters, shorter training times, and faster inference times. Photoroom’s AI models are specifically designed for e-commerce photography, ensuring precise tasks such as removing product image backgrounds, creating product catalog images, and generating high-performance advertising images. By focusing on specialized AI models, companies can minimize energy consumption while improving the quality of results for specific tasks.
Improving the quality of results:
It is common for generative AI users to make multiple requests to get the desired result. Reducing execution time at the query level can therefore seem ineffective if the user needs to make 20 prompts to get the expected result. An effective way to counterbalance this effect is to attempt to generate the desired result with a minimum number of generations. By designing more relevant and efficient models, AI companies can minimize energy consumption on a larger scale than the individual query, thus reducing the total number of queries and overall energy consumption.
The rebound effect: a blessing or a curse?
The rebound effect occurs when a resource made more accessible leads to greater adoption and overall higher consumption. The fast-fashion phenomenon is a perfect example: the emergence of low-cost clothing has led to excessive consumption. As AI becomes more efficient and accessible, it is expected that increased use will drive higher demand for resources. We have observed this at Photoroom: a massive adoption followed our optimization efforts.
The rebound effect is often viewed negatively, but in the context of e-commerce, Photoroom enables customers to replace energy-intensive and carbon-heavy practices with AI-powered image editing. For instance, practices like flying entire teams for photoshoots, transporting goods to Romania for shoots, or outsourcing Photoshop editing to agencies in India are now avoided, reducing both energy consumption and carbon emissions. Improving clothing photos with Photoroom allowed the British Red Cross to sell their donated clothing at a 13% higher price. In the restaurant industry, instead of cooking entire menus for photoshoots, businesses can take photos of the dishes directly during service, thus avoiding food waste.
The Jevons Paradox refers to a phenomenon where an efficiency gain leads to an increase in consumption greater than 100%. When the use of AI replaces a carbon-heavy workflow or improves an already sustainable workflow, it seems legitimate to ask whether this paradox has been reached or not for each use case.
Opportunities and the path to a sustainable AI ecosystem
Sustainability in AI represents a win-win-win opportunity for businesses. By focusing on improving the user experience, reducing costs, and minimizing environmental impact, AI companies can unlock significant value for themselves and their clients.
The broader AI ecosystem plays a critical role in sustainability, and all actors — cloud providers, developers, and end-users — can consider sustainable criteria when selecting their providers and partners, such as renewable energy use, low energy consumption, and a commitment to minimizing environmental impact.
A sustainable future in AI will require more responsible collaboration across the ecosystem.
Learn more about
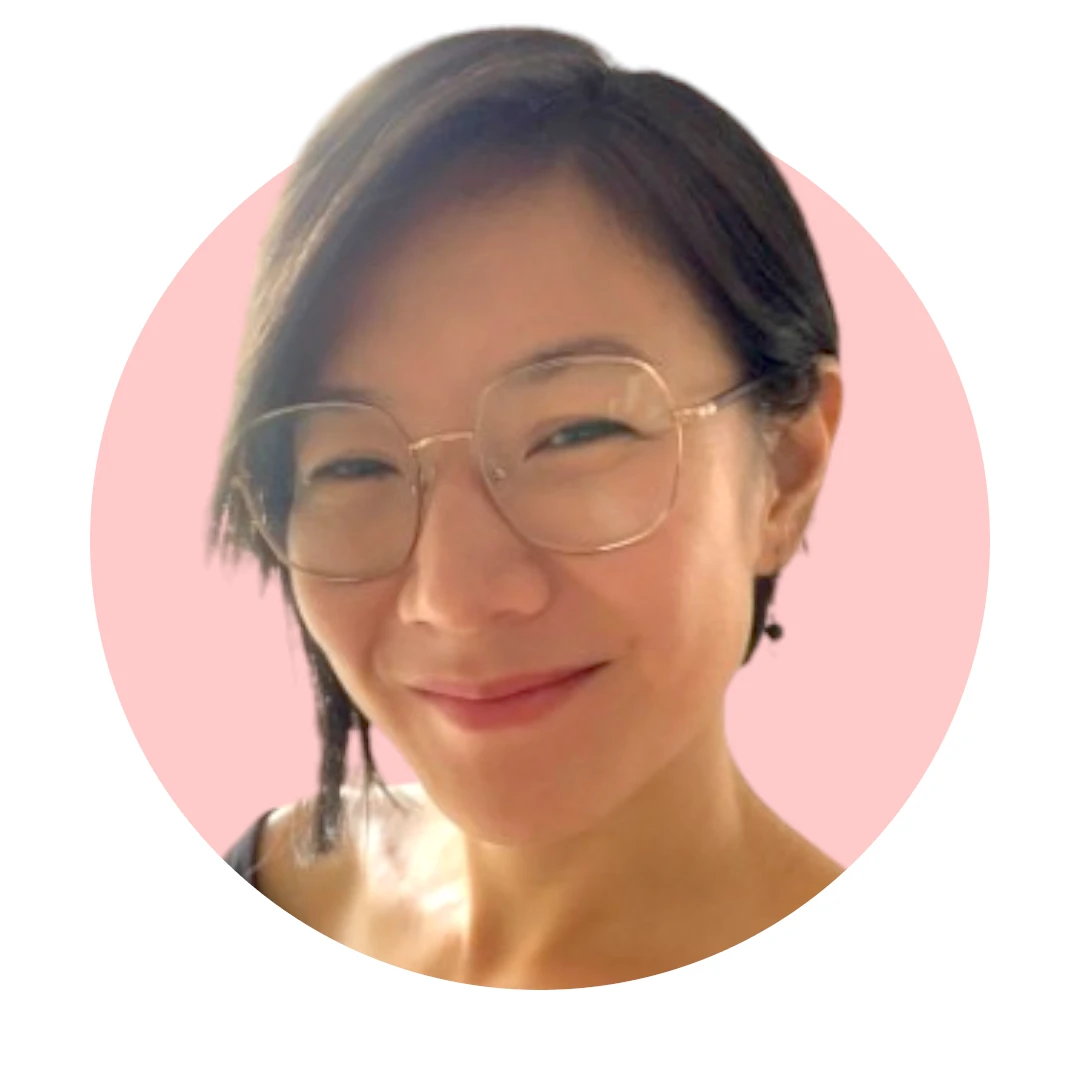
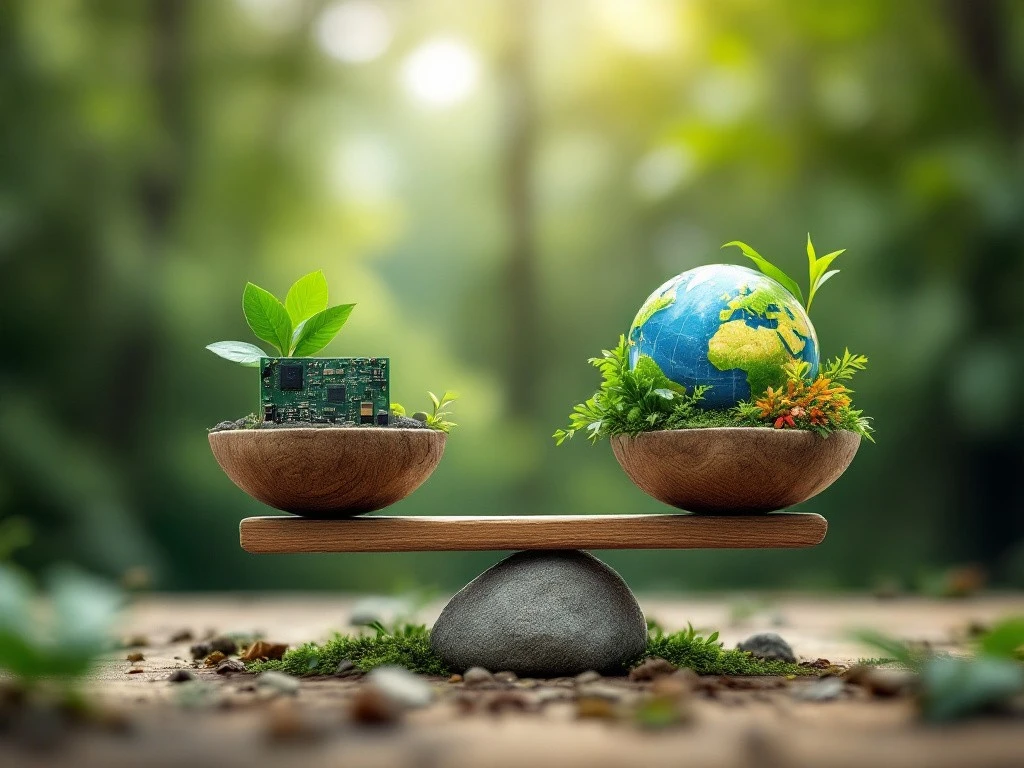
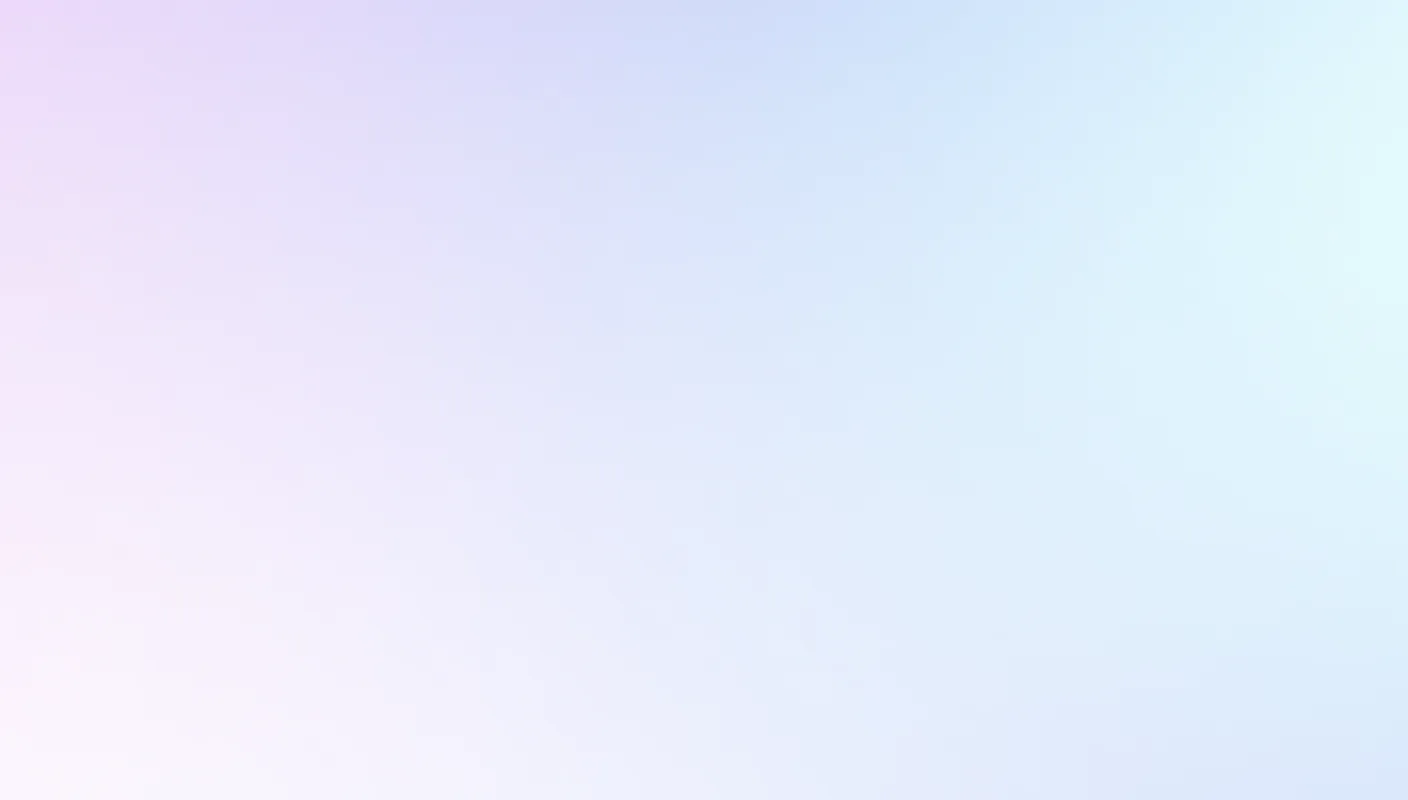
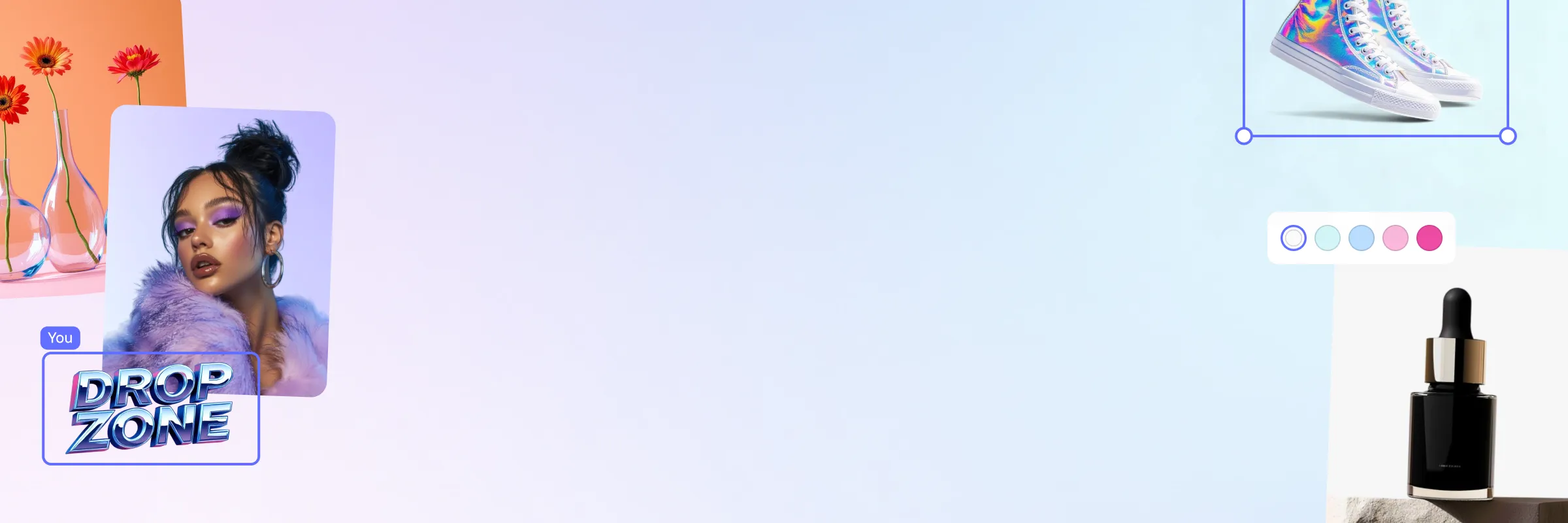
Design your next great image
Whether you're selling, promoting, or posting, bring your idea to life with a design that stands out.